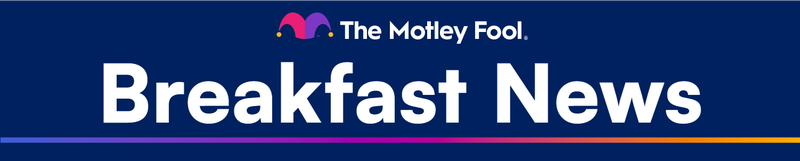
Breakfast News: Shakeups In The C-Suite
December 26, 2024
S&P 500 6,040 (+1.1%) |
|
Nasdaq 20,031 (+1.35%) |
|
Dow 43,297 (+0.91%) |
|
Bitcoin $98,550 (+0.23%) |

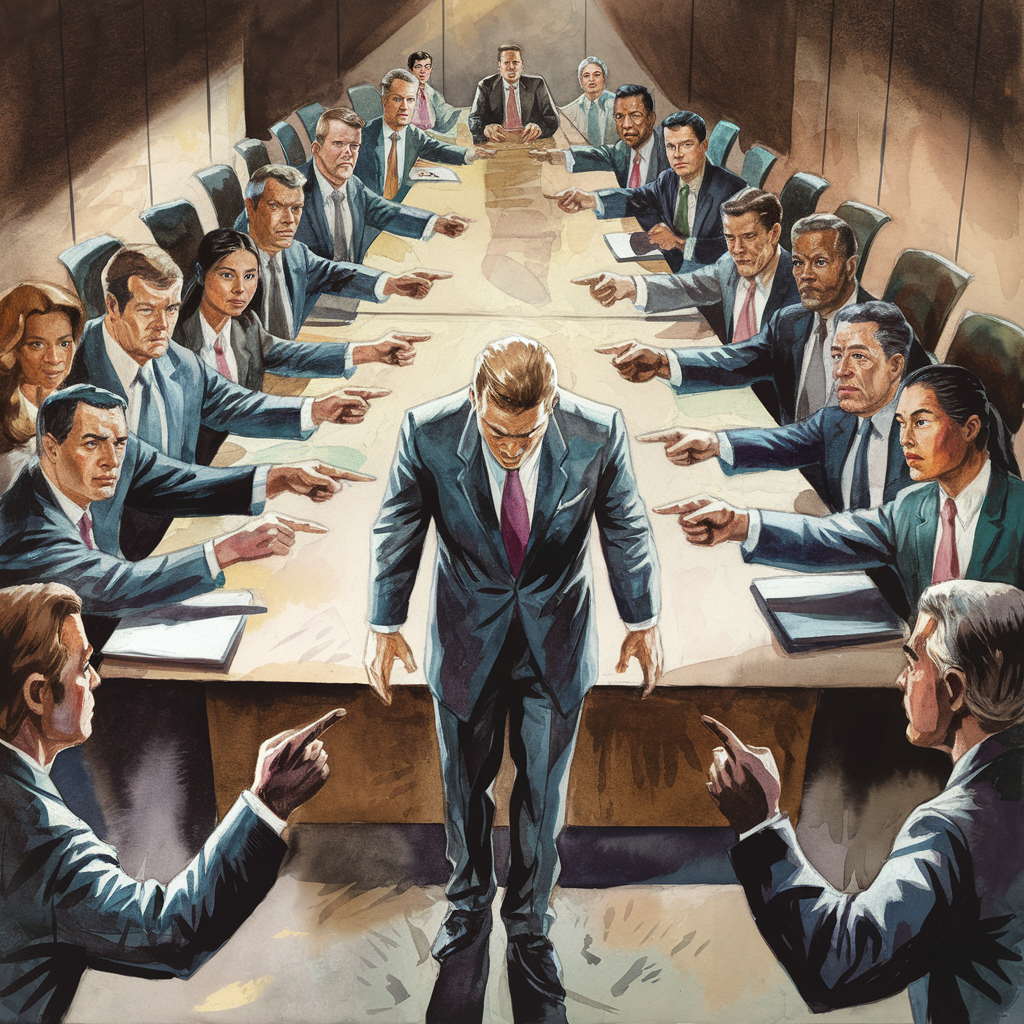
Source: Image created by JesterAI.
Patience is running short in the boardroom. While markets soar to new heights, companies left behind face mounting pressure for change. 2023 saw the highest rate of CEO turnover in a decade. So far, 2024 is on pace to eclipse that record.
Historic Turnover
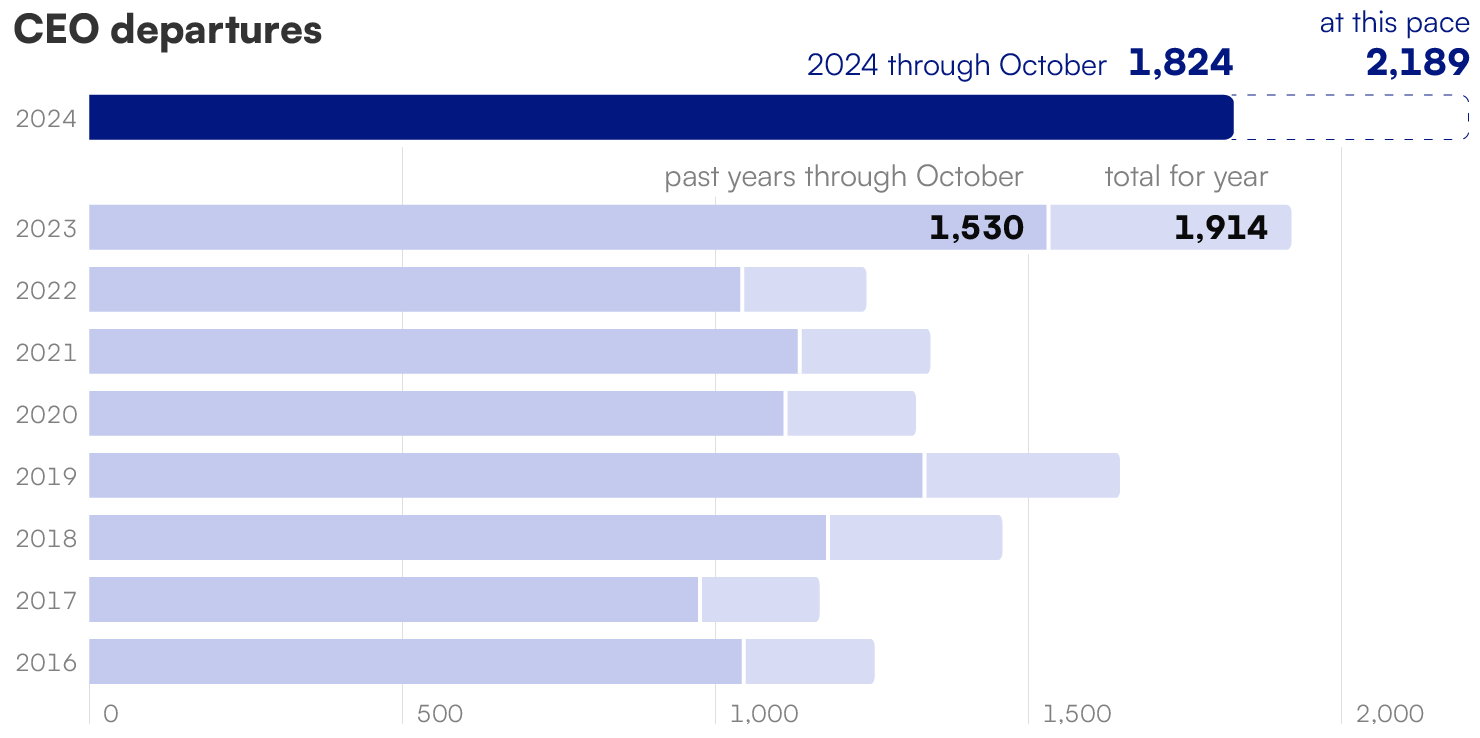
Looking Ahead: Matt Argersinger’s Take
It’s a Tough Gig
The scrutiny only gets more intense during bull markets like the current one. A CEO whose stock price doesn’t keep up with the runaway train of the market is a CEO with a short lifespan. Now, layer on the more modern investor demands for consistent revenue growth, higher profit margins, a global presence, and, yep, the pressure to somehow incorporate AI into every business process or product expression (or, at the very least, mention it a few dozen times during every conference call), and you can understand why CEO tenures are shorter than ever.
AI Spending Soars
Tech giants bet unprecedented sums on AI in 2024. Meta Platforms (META -5.00%), Alphabet (GOOG -3.21%), Amazon (AMZN -3.92%), and Microsoft (MSFT -3.58%) are projected to invest a combined $200 billion in AI initiatives this year, an outlay that has helped push big tech’s capital expenditure 56% higher through the first three quarters compared to 2023.
The spending spree focused on two critical areas: computing power and infrastructure. Meta’s early 2024 commitment to purchase 350,000 of Nvidia’s (NVDA -7.03%) high-end GPUs — each costing tens of thousands of dollars — exemplified the race for processing capacity. Meanwhile, data center spending is expected to reach $293 billion this year according to Gartner (IT -5.33%), driven primarily by generative AI preparations.
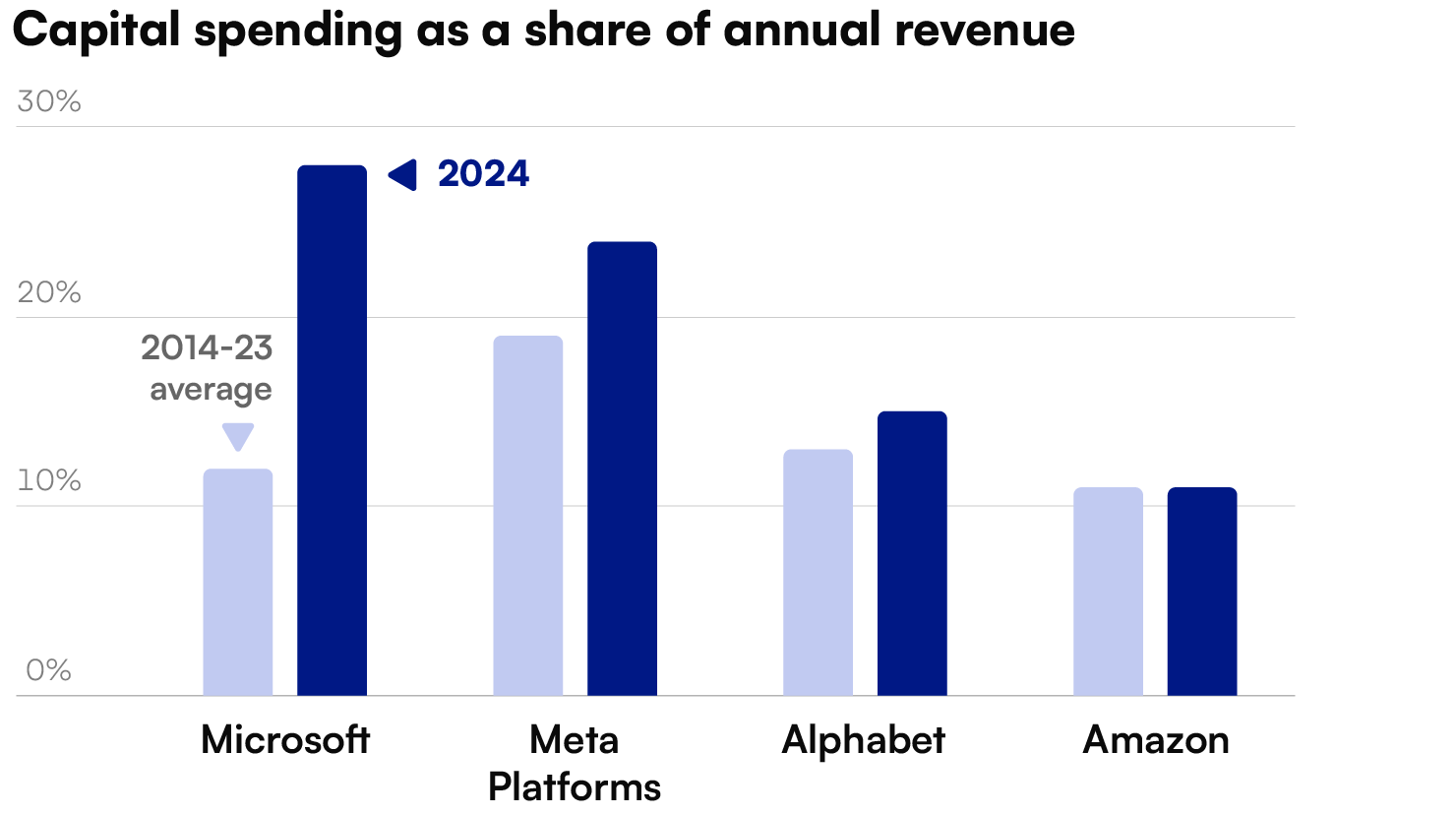
Looking Ahead: Asit Sharma’s Take
Hyperscalers Create Their Own GPUs
A growing trend that we’ll see as a "headline" for AI spend is the difference in computing power (and cost) required to train large language models, and the computation required to provide model outputs. Training is expensive: expect Nvidia to still dominate when it comes to providing GPU accelerators that train models with parameter counts in the trillions (i.e. really, really big data training sets). But we may also see a variety of new solutions and GPU/chip configurations that make inference cheaper.
Foolish Fun
How did your investment portfolio perform this year compared to your expectations? Looking back, would you have approached any of your investment decisions differently? Discuss with friends and family, or become a member to hear what your fellow Fools are saying!